GOTO is a vendor independent international software development conference with more that 90 top speaker and 1300 attendees. The conference cover topics such as .Net, Java, Open Source, Agile, Architecture and Design, Web, Cloud, New Languages and Processes
The Big Data Value Continuum
Orginally a blog post by Scott Jarr, Co-Founder & Chief Strategy Officer at VoltDB
Technology markets are challenging enough to understand but, when you throw in the added noise that typically accompanies early markets, gaining real insights can be next to impossible. It is not unusual to have ten or more vendors in a particular segment and adjacent segments, and countless products attempting to solve similar customer problems. Needless to say, tech markets are rarely clear in the beginning.
Big Data is just such a market today. One of the challenges we face at VoltDB is making sense of this market and understanding where we fit. This, of course, demands that we grasp the fundamental nature of the market and understand where others fit as well.
The following post is the first of a two part series that provides commentary to a couple of slides we recently built that aim to articulate the current state of the Big Data market. This discussion has already generated some very interesting dialog, and I’d welcome your inputs as well.
Part 1: The New Nature of Data
- Data exists on a time continuum. Data is no longer stationary. It moves from function to function within an organization. Gone are the days where a piece of information arrives, gets locked away in an Oracle (or DB2, MSSQL, etc.) system and is left to grow old and cold. Big Data is all about leveraging information for its total worth, and that means extracting the maximum value from data at various points in its life.
- The "things" we do with data are strongly correlated to its age. In the graphic below, we have represented time as the horizontal axis. To the far left is the point at which data is created.
- The value of data changes from the individual item to the aggregate over this time line. Now that we have an understanding of how we use data over a timeline, let’s use a real world example to demonstrate how the value of that data changes over the same timeline.
Just after data is created, it is highly interactive. We want to perform high velocity operations on that data at this stage – how fast can we place a trade, or serve an ad, or inspect a record?
Shortly after creation, we are often interested in a specific data instance relative to other data that has also arrived recently – how is my network traffic trending, what is my composite risk by trading desk, what is the current state of my online game leader board? Queries like these on high velocity data are commonly referred to as realtime analytics.
As data begins to age, our interest often changes from "hot" analytics to record-level storage and retrieval – store this URL, retrieve this user profile, etc. Write it to the database and read it back as necessary, but make sure those reads can be serviced very quickly because there’s often a user waiting for that data on the other side of a browser.
Can you detect the beginning of a trend? As data begins to age, its value does not diminish, but the nature of that value does begin to change.
Ultimately data becomes useful in a historical context. Organizations have found countless ways to gain valuable insights – trends, patterns, anomalies – from data over long timelines. Business intelligence, reporting, back testing are all examples of what we do to extract value from historical data. Additionally, we are now seeing an increasing use of data science – applications that explore data for increasingly deeper insights – not just observing trends, but discovering them. I call this Exploratory analytics.
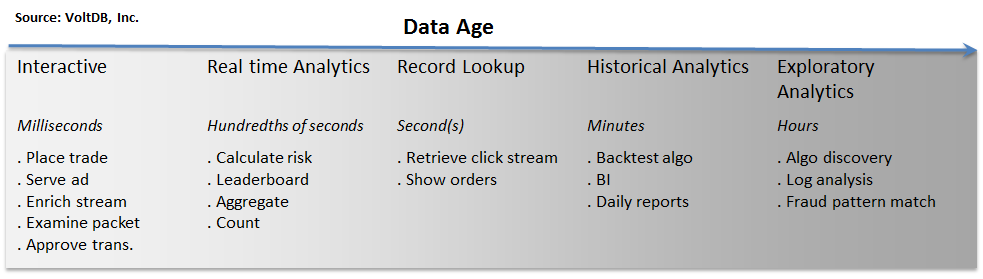
When I buy my new iPad, that individual transaction (data item) is highly valuable – to me, to Apple and to American Express. Over the course of a week, Apple may use that item with other near term items for realtime analytics – inventory control perhaps or regional trending. But the value of that individual item will rapidly decline. In 6 months, there will be almost no value in knowing that I bought my iPad in the Boston Apple store on March 17th.
However, there will be tremendous value to Apple when my purchase is looked at relative to many others like me over a six-month period – that is, the value of data is high in the aggregate. Finding people of a similar cohort, who buy additional products or services, are valuable insights for Apple to use to sell additional products to large consumer populations (something Apple is very good at doing). Over time, the value of that one data item (my iPad purchase) is now exclusively in its relationship to many other related purchases.
So, as we’ve talked here in Part 1, data is no longer purely static. It lives on a continuum where different actions are taken on it and value is derived from it in different ways. This is the promise of Big Data.
Clearly, we can’t use a single datastore to service our needs across the entire continuum. In part 2, I’ll overlay popular database technologies onto this continuum to illustrate which solutions fit best for different kinds of Big Data needs.
What do you think?
Prior to joining VoltDB, Scott was VP Product Management and Marketing at on-line backup SaaS leader LiveVault Corporation. While at LiveVault, Scott was key in growing the recurring revenue business to 2000 customers strong, leading to an acquisition by Iron Mountain. Scott has also served as board member and advisor to other early-stage companies in the search, mobile, security, storage and virtualization markets.
Scott has an undergraduate degree in mathematical programming from the University of Tampa and an MBA from the University of South Florida.